Table of Contents
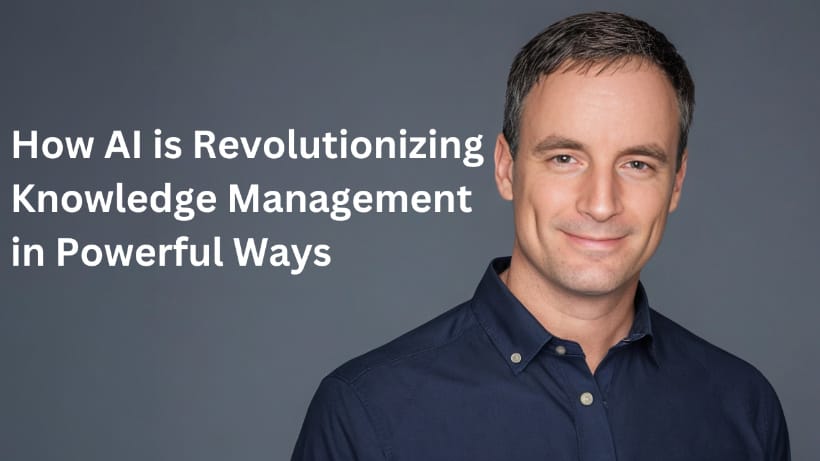
Having spent years in Knowledge Management (KM) at a global Fortune 500 investment bank, I’ve witnessed the profound impact that a robust Knowledge Management (KM) system can have on an organization. By authoring over 1500 knowledge articles tailored for both non-technical users and technical support staff, I helped drive a remarkable 1000% increase in monthly article views, significantly reducing helpdesk calls and empowering users to solve their own problems. The metrics were clear: a well-structured knowledge base enhances productivity and user satisfaction across the organization.
However, as Artificial Intelligence (AI) continues to evolve, a new question emerges: What is the future of Knowledge Management in an AI-driven world? The rapid advancements in AI, especially with tools like Large Language Models (LLMs), have sparked concerns about the potential obsolescence of roles such as Knowledge Managers, technical writers, and librarians.
Yet, I believe this fear is misplaced. Instead of viewing AI as a replacement for KM, we should see it as a powerful extension of it. At its core, AI Knowledge Management represents the integration of AI’s capabilities with traditional KM practices. It’s about using AI to scale knowledge creation, improve accuracy, and ultimately enhance the user experience. In this article, I’ll explore how AI is reshaping the landscape of Knowledge Management, why human oversight remains vital, and what the future holds for this evolving field.
The Evolution of Knowledge Management: From Traditional to AI-Enhanced
The concept of Knowledge Management has evolved significantly over the past few decades. Traditionally, KM focused on creating, storing, and disseminating information to help users solve problems and make informed decisions. In my role, I implemented a KM strategy that involved writing and maintaining thousands of articles, from detailed troubleshooting guides to quick-reference FAQs. The impact was undeniable: approximately 70% of visits to the self-service portal resulted in users finding solutions without raising an incident with the IT Service Desk.
Challenges of Traditional Knowledge Management
Despite these successes, traditional KM is not without its challenges:
- Maintenance Overload: As the knowledge base grows, maintaining the accuracy and relevance of each article becomes increasingly complex. In my experience, once the knowledge base exceeded 1,000 articles, the effort required to keep it up to date became a significant burden.
- Distributed Authorship Issues: Ideally, KM should involve distributed authorship, where Subject Matter Experts (SMEs) from various departments contribute to the knowledge base. However, this often results in inconsistencies in quality, as SMEs may not have the writing expertise or time needed to create comprehensive documentation (or the desire to do so).
- User Accessibility and Searchability: Even with a well-organized knowledge base, users can struggle to find the information they need, especially when searching for niche or specific topics.
These issues highlight a gap that AI can effectively fill. Enter AI-powered Knowledge Management: a paradigm shift that enhances traditional KM by automating knowledge creation, improving search functionality, and optimizing content delivery.
AI as an Extension of Knowledge Management
In many ways, AI Knowledge Management is a natural evolution of traditional KM practices. AI can automate the creation of knowledge articles, analyze user queries to improve search results, and even predict the kind of information users might need based on their behavior. However, the idea that AI will fully replace Knowledge Managers is a misconception.
AI, particularly in its current form, is limited by the data it has been trained on. Generative AI models, like those used in chatbots or content creation tools, are designed to generate responses based on patterns in existing data. While these models can be incredibly useful in creating initial drafts or answering common queries, they often lack the contextual understanding required for specific, nuanced situations. For example, in a bespoke application environment—common in large enterprises—AI may struggle to provide accurate answers because its training data is based on more standardized implementations.
This is where the AI-augmented Knowledge Manager comes into play. Instead of being replaced, Knowledge Managers can leverage AI to enhance their capabilities. AI can handle repetitive tasks like updating FAQs or generating basic troubleshooting guides, freeing up Knowledge Managers to focus on higher-level activities like content verification, complex problem-solving, and strategic planning. By acting as the human gatekeeper, the Knowledge Manager ensures that the knowledge generated by AI is accurate, relevant, and fit for purpose.
In the next section, we’ll delve deeper into how generative AI can support the creation of knowledge and why human oversight is still a critical component of effective Knowledge Management.
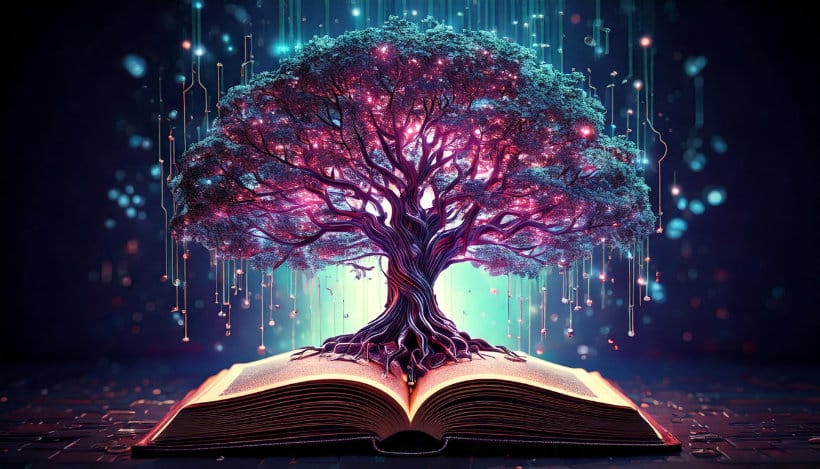
AI as an Extension, Not a Replacement, of Knowledge Management
The integration of Artificial Intelligence into Knowledge Management has sparked fears that roles like Knowledge Managers, technical writers, and librarians may become obsolete. However, this perspective is only partially correct. While AI has the potential to automate many aspects of knowledge creation and maintenance, it still requires human oversight to ensure accuracy, relevance, and context. Rather than seeing AI as a replacement, we should view it as a powerful tool that enhances traditional Knowledge Management practices.
The Misconception of AI Replacing Knowledge Managers
At its core, AI is a sophisticated tool for processing and managing information. Large Language Models (LLMs) and other AI systems are trained on vast datasets, learning patterns and relationships in text data to generate responses, articles, and insights. This capability has led some to believe that AI can fully take over the role of Knowledge Managers. However, the reality is more nuanced.
Generative AI models excel in creating content based on existing knowledge, but they lack the deep contextual understanding that a human Knowledge Manager brings to the table. For instance:
- Hallucinations: AI models can generate confident yet incorrect answers, known as hallucinations. This happens because the AI makes statistical guesses based on the patterns it has learned, rather than drawing from a true understanding of the topic.
- Bias in Training Data: AI models are trained on a wide variety of sources, which can introduce biases, particularly when dealing with bespoke or niche implementations within an organization. For example, an LLM trained primarily on publicly available software documentation may struggle to address the unique configurations and customizations of enterprise-level applications.
In many large organizations, including those I’ve worked with, knowledge is deeply tied to specific processes, tools, and environments that are not part of the typical training data for AI models. This makes it challenging for AI to accurately generate or update knowledge without human input. Here, the role of the AI-augmented Knowledge Manager becomes crucial. By working alongside AI, Knowledge Managers can:
- Curate and Validate Content: AI can draft initial versions of knowledge articles, but a Knowledge Manager is needed to verify the content, ensuring it aligns with the organization’s specific needs.
- Provide Contextual Adjustments: A human expert can tailor AI-generated content to fit the unique requirements of bespoke systems or processes that the AI may not fully understand.
- Maintain Data Integrity: Continuous monitoring and updating by human gatekeepers help prevent outdated or incorrect information from spreading, which could degrade the overall quality of the knowledge base.
Leveraging AI for Scalable Knowledge Creation
One of the most significant advantages of integrating AI into Knowledge Management is its ability to scale content creation quickly. In a traditional KM setup, creating and updating knowledge articles is often a time-consuming task, especially when dealing with complex applications or frequent software updates. AI can help accelerate this process by:
- Generating Drafts of Knowledge Articles: Using natural language processing, AI can create drafts of troubleshooting guides, FAQs, and how-to articles based on input from existing documentation, release notes, and ticketing systems like JIRA.
- Analyzing User Queries: By examining patterns in user search queries and feedback, AI can identify gaps in the current knowledge base and suggest new topics for articles, ensuring that the content remains relevant and comprehensive.
- Updating Existing Documentation: AI can be trained on existing documentation and release notes to automatically update articles when new features are added or when software changes occur.
This scalable approach allows organizations to keep their knowledge bases up to date without significantly increasing the workload for Knowledge Managers. Instead, Knowledge Managers can focus on higher-value tasks, such as refining content quality, providing strategic insights, and enhancing the user experience.
The Role of Generative AI in Knowledge Creation
Generative AI has the potential to revolutionize Knowledge Management by automating various aspects of content creation. In practical terms, this means using AI to generate knowledge articles, product demos, user guides, and FAQs at a much faster pace than a human team could achieve alone. However, the real challenge lies in ensuring the quality and accuracy of this content, especially in specialized or bespoke environments.
Real-World Applications of Generative AI in KM
Consider a scenario in a large enterprise with a complex IT landscape. When a new software feature is rolled out, traditional KM processes might involve gathering input from SMEs, documenting the changes, and updating relevant articles in the knowledge base. This process can take weeks, especially if the updates are extensive.
With AI-powered Knowledge Management, this process can be streamlined significantly:
- Training the Model on Existing Data: By training an AI model on existing documentation, ticketing systems like JIRA, and other sources, it gains an understanding of the organization’s specific knowledge.
- Generating Initial Drafts: Once the new feature details are input into the system (e.g., through release notes), the AI can generate initial drafts of knowledge articles, complete with step-by-step instructions, screenshots, and even video demonstrations if the model has access to visual content.
- Human Review and Refinement: The drafts are then reviewed by Knowledge Managers, who verify the accuracy, add contextual details, and make any necessary adjustments before publishing.
This approach not only speeds up the documentation process but also enhances the quality and consistency of the content. The AI learns from each iteration, improving its understanding and output over time.
Overcoming the Challenges of Bespoke Environments
A significant limitation of generative AI in Knowledge Management is its reliance on training data. In bespoke environments—where applications and processes are highly customized—standard AI models may produce inaccurate or misleading content because they are trained on generalized data. However, organizations can overcome this challenge by:
- Developing Organization-Specific Models: Training AI models on internal data sources, such as historical knowledge articles, system logs, and user feedback, helps create a more tailored solution that better understands the nuances of the organization.
- Continuous Learning and Feedback Loops: Implementing a feedback loop where users and Knowledge Managers can correct and refine the AI’s output ensures that the model continuously improves in accuracy and relevance.
The role of the human Knowledge Manager remains pivotal in this process. By guiding the AI and providing critical feedback, Knowledge Managers ensure that the knowledge base remains accurate, comprehensive, and fit for purpose.
In the next section, we’ll explore how AI is transforming user interactions with knowledge, shifting from passive consumption to proactive, personalized support.
Active vs. Passive Knowledge Delivery: The Shift in User Interaction
In traditional Knowledge Management (KM), users typically engage with a passive self-service model: they encounter a problem, search the knowledge base, and read articles or watch videos to find solutions. While this approach has been effective in many organizations, it often relies on users actively seeking information. However, the landscape is rapidly changing with the integration of AI-powered Knowledge Management systems, which are shifting towards a more active and interactive user experience.
The Limitations of Passive Self-Service
Passive self-service has its benefits, particularly when it comes to empowering users to resolve their own issues. During my time managing a KM system at a major financial institution, we saw a 30% increase in self-service utilization thanks to a robust knowledge base. However, even with comprehensive articles and a well-structured search system, the passive approach often left gaps:
- Search Friction: Users might not know the right keywords to use, leading to frustration when searching for specific topics.
- Information Overload: With a large number of articles available, users can easily become overwhelmed by the volume of information, struggling to find the most relevant content.
- Delayed Resolution: Users must navigate through multiple articles to piece together a solution, which can slow down problem resolution.
To address these challenges, AI Knowledge Management systems are evolving from passive repositories to more active, dynamic solutions that anticipate user needs and provide personalized assistance in real-time.
The Rise of Active, AI-Driven Knowledge Delivery
With advancements in AI, we are witnessing a shift towards active knowledge delivery, where systems proactively assist users, anticipating their needs based on context and behavior. Here’s how AI could transform the user experience in KM:
- Contextual Assistance and Proactive Support
- Predictive Insights: By analyzing user behavior, AI can predict the type of support a user might need even before they initiate a search. For example, if a user is repeatedly encountering an error while using an application, the system can proactively suggest relevant troubleshooting guides or FAQs.
- Chatbots and Virtual Assistants: AI-powered chatbots can engage users directly, asking targeted questions to understand their issues better and guiding them to the most relevant solutions. Unlike traditional search functions, chatbots can deliver a more interactive and conversational experience, mimicking a human support agent’s approach.
- In-Application Guidance: AI can also integrate directly into applications, offering real-time assistance as users navigate features. For instance, if a user is configuring a complex setting in a software application, the system might display a tooltip or a step-by-step guide based on common user queries related to that feature.
- Personalized Knowledge Recommendations
- Dynamic Content Delivery: Rather than relying on users to search the knowledge base, AI can deliver personalized content recommendations based on the user’s role, previous interactions, and current activities. For example, a project manager might receive updates on relevant new features or common troubleshooting tips based on the tools they frequently use.
- Learning from User Feedback: AI systems can continuously learn from user interactions and feedback, refining their recommendations. If users consistently find certain articles helpful or rate specific responses positively, the system can prioritize these resources for similar queries in the future.
This active approach not only improves user satisfaction by providing quicker and more accurate solutions but also significantly enhances productivity by reducing the time spent searching for information.
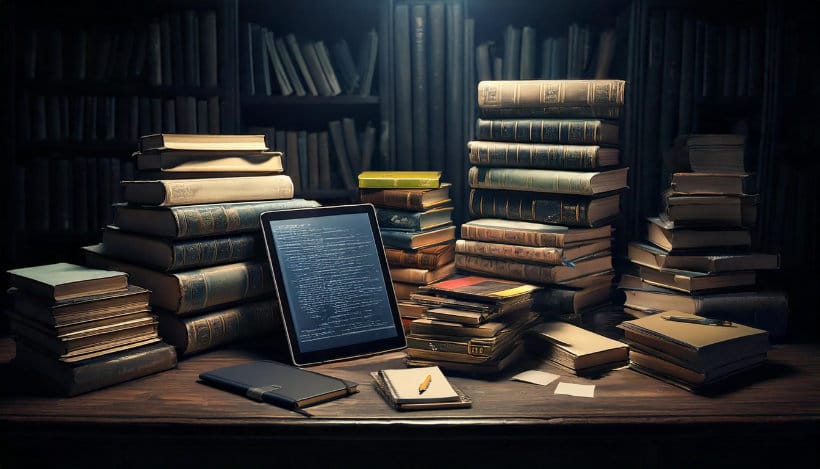
The Human Element: Why Knowledge Managers Are Still Vital
As AI takes on a more prominent role in Knowledge Management, it’s easy to assume that the need for human Knowledge Managers will diminish. However, my experience suggests otherwise. Even as AI tools automate many aspects of KM, the human element remains critical in maintaining the quality and relevance of the knowledge base.
The Role of the Knowledge Manager in an AI-Driven World
Knowledge Managers bring a unique blend of skills that AI cannot fully replicate, including deep contextual understanding, critical thinking, and the ability to empathize with end users. Here are key areas where human oversight is indispensable:
- Quality Assurance and Verification
- Content Accuracy: AI-generated content, while useful, is not infallible. Hallucinations—instances where the AI confidently generates incorrect information—are a known issue. Knowledge Managers play a crucial role in vetting and verifying this content, ensuring it aligns with the organization’s specific needs and is factually correct.
- Contextual Adaptation: AI might generate generic responses based on its training data, but Knowledge Managers provide the necessary context and adjustments. For example, a generic troubleshooting guide might need customization to reflect specific configurations or workflows unique to the organization.
- Curating and Updating Knowledge
- Strategic Content Curation: Knowledge Managers have a strategic understanding of the knowledge needs of their organization. They can identify gaps in the knowledge base, prioritize content updates, and decide which new articles or resources should be developed based on emerging user needs.
- Continuous Improvement: By analyzing user feedback, search patterns, and chatbot interactions, Knowledge Managers can refine and enhance the knowledge base, ensuring it evolves with changing business requirements.
- Training and Enhancing AI Models
- Input for Model Training: As organizations develop bespoke AI models tailored to their specific needs, Knowledge Managers are invaluable in providing input data, refining prompts, and fine-tuning the model’s outputs. Their expertise ensures that the AI model’s responses are aligned with the organization’s terminology, processes, and standards.
- Monitoring AI Performance: Knowledge Managers can track the performance of AI-powered tools, identifying areas where the AI might be underperforming or generating incorrect responses. This feedback loop is essential for continuous improvement.
The role of the Knowledge Manager is thus shifting from a sole content creator to a strategic overseer and curator, leveraging AI as a powerful tool while maintaining human oversight to ensure quality and relevance.
Predictions for the Future of KM in an AI-Driven World
Looking ahead, the integration of AI in Knowledge Management will continue to transform the field, moving us closer to a future where AI handles routine, repetitive tasks, allowing human experts to focus on strategic and complex problems. Here are some predictions on how AI-powered Knowledge Management might evolve:
- Greater Personalization and Automation: AI models will become more adept at understanding user intent and context, providing highly personalized and automated support. We might see a rise in self-healing systems, where AI not only identifies issues but also resolves them autonomously without user intervention.
- AI as a Knowledge Creator: As AI models advance, particularly with the potential development of Artificial General Intelligence (AGI), they may start to generate “first-source” knowledge—making discoveries or generating insights that were not part of their training data. This could revolutionize the way we think about KM, shifting from managing existing knowledge to facilitating the creation of new knowledge.
- Increased Focus on Human-AI Collaboration: The future of KM will likely involve a closer partnership between AI systems and human Knowledge Managers. The human role will focus on strategic decision-making, ethical considerations, and ensuring the AI remains aligned with the organization’s goals and values.
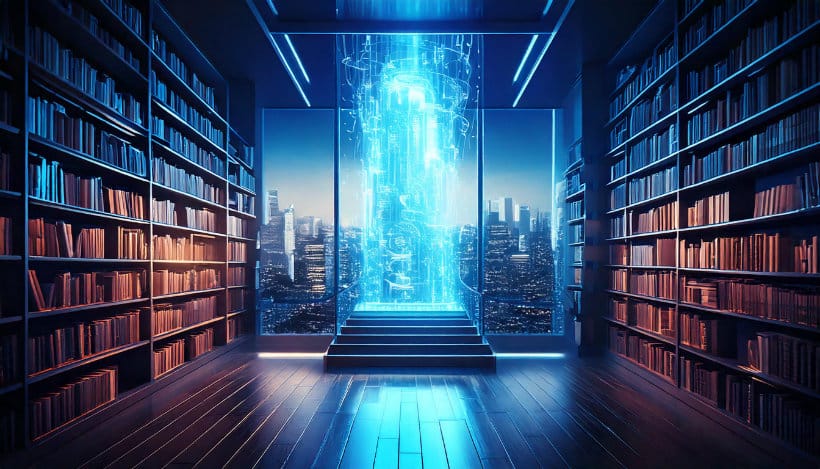
Conclusion: The Future of Knowledge Management
The future of Knowledge Management in an AI-driven world is not about choosing between humans and AI but about leveraging the strengths of both. AI Knowledge Management offers incredible potential for scaling content creation, enhancing user interactions, and streamlining information delivery. However, the expertise, critical thinking, and contextual understanding of human Knowledge Managers are irreplaceable in maintaining the quality and integrity of the knowledge base.
As AI continues to evolve, so too must the role of the Knowledge Manager. By embracing AI as a powerful tool rather than a threat, Knowledge Managers can enhance their capabilities, driving more effective and efficient knowledge solutions that meet the needs of today’s fast-paced digital environments.
Note: AI tools supported the brainstorming, drafting, and refinement of this article.
Jacob is a seasoned IT professional with 20+ years of experience and a proven track record of driving business value in the financial services sector. His extensive expertise spans Business Analysis, Knowledge Management, and Solution Architecture. Skilled in UX/UI design and rapid prototyping, he leverages comprehensive experience with ServiceNow and ITSM competencies. Jacob’s passion for AI is reflected in his Azure AI Engineer Associate certification.